Building a Next.js App with AWS Amplify, Lambda, and OpenAI API
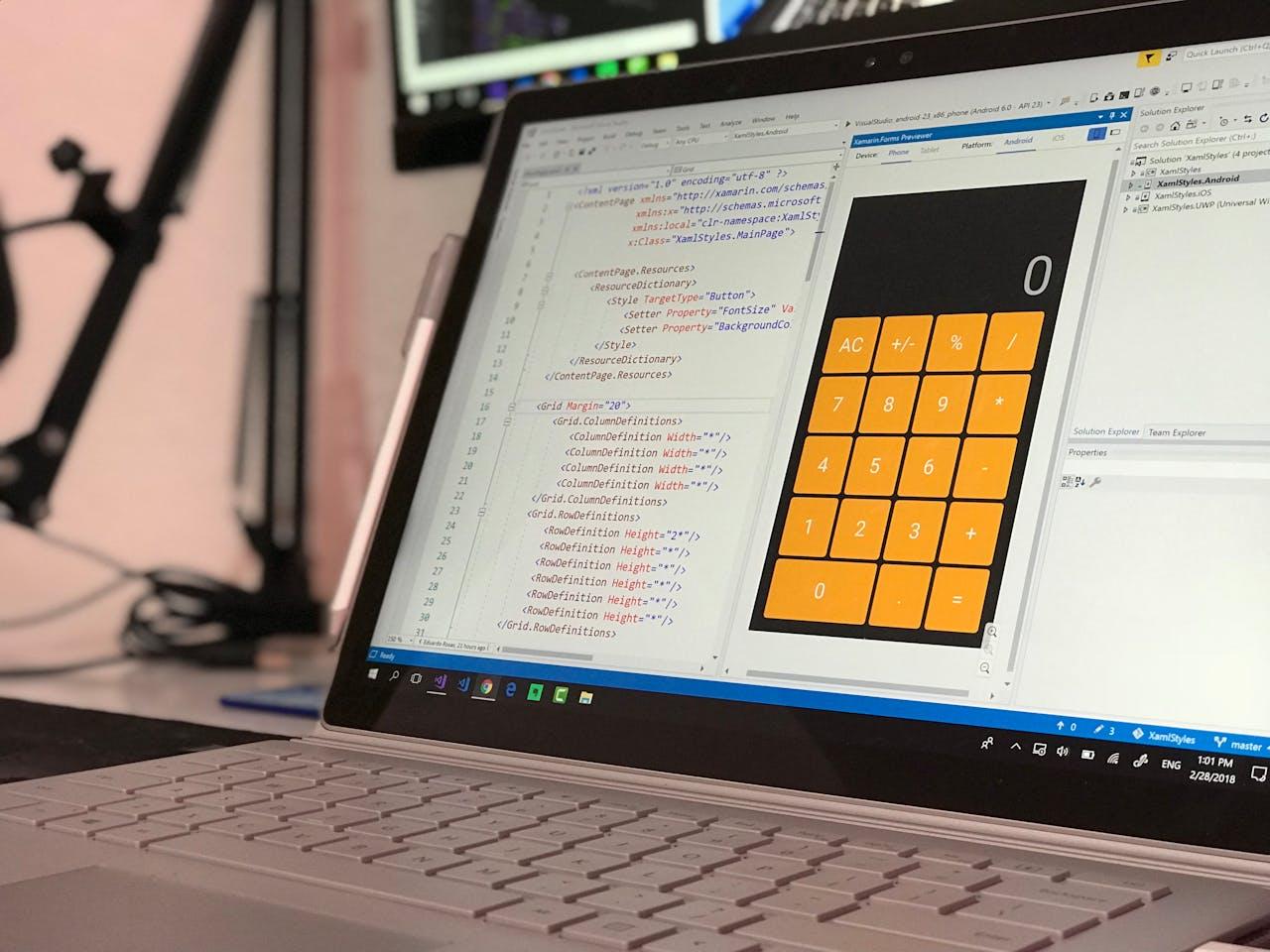
Table Of Content
By CamelEdge
Updated on Tue Jul 30 2024
Introduction
In this guide, we will build a simple web app that fetches and displays a random quotation using the OpenAI API. The app will run on AWS Amplify, with a backend powered by AWS Lambda for the OpenAI API calls. The Lambda function will be written in Python and will require external libraries, so we will also set up a Lambda layer. Let's get started.
Prerequisites
- AWS Account: Open an account on AWS.
- Configure AWS for Local Development: Follow this guide to set up AWS for local development.
- Node.js: Install Node.js if it is not already installed.
Step 1: Create a Next.js App
To create a Next.js app, open the terminal, cd
into the directory you would like to create the app in and run the following command
npx create-next-app@latest my-nextjs-app
You can use a template through the --example
flag.
Navigate into the new directory:
cd my-nextjs-app
and start the development server:
npm run dev
Open http://localhost:3000
in your browser to see your Next.js app.
Step 2: Deploy to AWS Amplify
Push your code to a repository using git push
. Follow this guide if you have not done this before.
Now deploy the app to AWS Amplify. Follow this guide
Step 3: Build and Connect the Backend
- Create a folder
amplify
in the root of your project directory. - Within the
amplify
folder, create the following subfolders:data
,functions
,lambda
, andlayer
. These folders will contain you backend resources.
Your app directory structure should look like this:
├── my-nextjs-app/
│ ├── amplify/
│ │ ├── data/
│ │ ├── functions/
│ │ ├── lambda/
│ │ └── layer/
│ ├── app/
│ │ ├── pages.tsx
│ │ └── layout.tsx
├── public/
├── node_modules/
├── .gitignore
├── package-lock.json
├── package.json
└── tsconfig.json
└── next.config.mjs
- Install the Amplify dependencies for building the backend:
npm add --save-dev @aws-amplify/backend@latest @aws-amplify/backend-cli@latest typescript
- Define the GraphQL schema using Amplify CLI's GraphQL library:
// amplify/data/resource.ts
import { type ClientSchema, a, defineData } from "@aws-amplify/backend";
import { quote } from "../functions/resource"
const schema = a.schema({
quote: a
.query()
.arguments({
name: a.string(),
})
.returns(a.string())
.handler(a.handler.function(quote))
.authorization(allow => [allow.authenticated()]),
})
export type Schema = ClientSchema<typeof schema>
export const data = defineData({
schema,
})
This defines a GraphQL query named quote
with:
- arguments: Accepts an argument named
name
of type string. - returns: Returns a string type.
- handler: The resolver function for this query is defined using
a.handler.function(quote)
. We will define the functionquote
next and it will contain the logic to fetch the data for this query. This function is powered by AWS Lambda.
- Using Amplify, we can write the resolver for the above query with a Lambda function handler in JavaScript. However, in this blog, we aim to write the Lambda function handler in Python. Therefore, we will initially create a dummy JavaScript function
quote
as required by the schema above. Later, we will replace it with the Python Lambda function and set it as the resolver.
//functions/handler.ts
import type { Schema } from "../data/resource"
export const handler: Schema["quote"]["functionHandler"] = async (event, context) => {
// your function code goes here
const {name} = event.arguments
return `Hello, ${name}!`;
};
Create this resource using:
// functions/resource.ts
import { defineFunction } from '@aws-amplify/backend';
export const quote = defineFunction({
// optionally specify a name for the Function (defaults to directory name)
name: 'quote',
// optionally specify a path to your handler (defaults to "./handler.ts")
entry: './handler.ts'
});
- Next, create the entry point for your backend, with the following code:
// backend.ts
import { defineBackend } from '@aws-amplify/backend';
import { data } from "./data/resource"
import { quote } from './functions/resource';
const backend = defineBackend({
data,
quote
})
You can now test the backend locally by running npx ampx sandbox
. This command will generate an amplify_outputs.json file, which your app can use to test the backend in an isolated cloud sandbox environment. This environment allows you to build, test, and iterate on your full-stack app quickly. You can also push your changes to GitHub for deployment.
However, there are additional steps to complete. We still need to create a Python-based Lambda function that will generate a random quote using the OpenAI LLM model. Additionally, we need to update the frontend of the app to display the generated quote.
Step 4: Python-based Lambda Function
Create a Python-based Lambda function
# lambda/hander.py
from openai import OpenAI
import json
client = OpenAI()
def handler(event, context):
name = event.get('arguments', {}).get('name')
response = client.chat.completions.create(
model="gpt-4o",
response_format={ "type": "json_object" },
messages=[
{"role": "system", "content": "You are a helpful assistant designed to output JSON."},
{"role": "user", "content": "Generate a new quotation."}
]
)
return f"Hello {name} \n" + json.loads(response.choices[0].message.content)['quote']
This handler function uses the OpenAI API to generate a quotation based on a prompt, then returns a personalized greeting with the generated quote.
Since we need the openai
library, we will create an AWS Lambda Layer to include it. First, create a folder named python
inside amplify/layer
. Add a file named requirements.txt
to amplify/layer
listing the required libraries— in this case, just openai. Then, run the following commands to install the dependencies.
python3 -m pip install -r requirements.txt --platform manylinux2014_x86_64 --target ./amplify/layer/python --python-version 3.12 --only-binary=:all:
This will install the required libraries in the folder amplify/layer/python
To deploy the handler and layer to AWS, we will use AWS CDK. Add the following code to the backend.ts
file:
//functions/backend.ts
...
const stack = backend.createStack("Mystack");
// - create a lambda layer
const python_layer = new lambda_.LayerVersion(stack, 'python_layer', {
removalPolicy: RemovalPolicy.DESTROY,
code: lambda_.Code.fromAsset("./amplify/layer/"),
compatibleRuntimes: [lambda_.Runtime.PYTHON_3_12]
})
// - create a lambda function
const quote_openai = new lambda_.Function(stack, "quote_openai", {
code: lambda_.Code.fromAsset("./amplify/lambda"),
runtime: lambda_.Runtime.PYTHON_3_12,
handler: "handler.handler",
layers: [python_layer],
timeout: Duration.seconds(100),
})
quote_openai.addEnvironment('OPENAI_API_KEY', process.env.OPENAI_API_KEY as string);
backend.data.addLambdaDataSource('quote_openai', quote_openai);
Step 5: Make frontend updates
Go to app/page.tsx
and add the following
//app/page.tsx
'use client'
import { generateClient } from "aws-amplify/api"
import type { Schema } from "@/amplify/data/resource"
import { Amplify } from 'aws-amplify';
import outputs from "../../amplify_outputs.json";
import { useState, useEffect } from "react";
Amplify.configure(outputs);
const client = generateClient<Schema>()
export default function App() {
const [message, setMessage] = useState('');
const fetchQuote = async () => {
const response = await client.queries.quote({
name: "CamelWdge",
})
if (response.data !== null && response.data !== undefined) {
setMessage(response.data);
} else {
console.error("No data received from query.");
}
};
useEffect( () => {
fetchQuote();
},[]);
return (
<h2>{message}</h2>
);
}
You can test it locally by running npx ampx sandbox
and starting the development server npm run dev
. Every time you visit http://localhost:3000 you will see a new personalized quotation.
Note You may need to change the Lambda resolver function via AWS AppSync console to point to the Python based function created above.